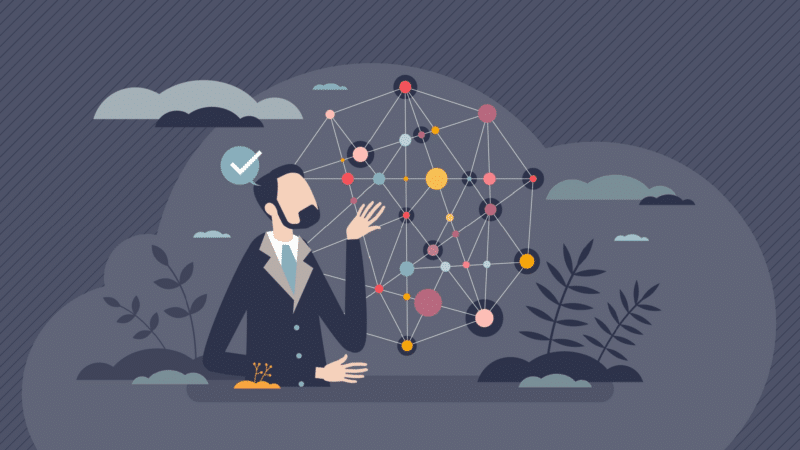
In an age where data is pivotal to business success, the transformative role of knowledge graphs in organizing and interpreting information cannot be overstated. Knowledge graphs serve as a bridge between raw data and meaningful insights, making them essential tools for industries such as search engines and digital marketing. While the term “knowledge graph” gained prominence after Google’s introduction in 2012, the underlying concepts date back to the 1980s, highlighting the long-standing need for systems that mimic human cognitive processes in handling interconnected information.
For professionals in fields like software development and digital marketing, the value of knowledge graphs lies in their ability to define relationships between entities, thereby enhancing information retrieval. Unfortunately, many practitioners still struggle to understand their functionality. Knowledge graphs provide a structured yet flexible approach to organizing information, allowing businesses to evolve from casual data collection to a more rigorous data management framework that can adapt as client bases grow and complexity increases.
Consider a digital marketing agency grappling with the chaos of unstructured client data. Initially, the agency may collect data haphazardly. However, with an expanding clientele, inconsistencies become more pronounced, leading to difficulties in data analysis. Introducing an ontology—a structured way to categorize and define relationships among information—helps establish a standard for data entries. Yet, while ontologies improve consistency, they still lag in managing nuanced queries related to client needs and tool efficacy.
The emergence of knowledge graphs addresses these challenges effectively. By structuring data into interconnected nodes (entities) and edges (relationships), supplemented by predicates that outline their interrelations, knowledge graphs empower professionals to traverse complex datasets. This semantic inference capability identifies patterns and insights that traditional databases may overlook, ultimately offering a more profound understanding of the underlying data landscape.
The article identifies key components of knowledge graphs—nodes, edges, and properties—while elucidating their capabilities, such as contextual inference and dynamic recommendations. This foundational knowledge allows businesses to derive actionable insights, fostering better decision-making strategies. As knowledge graphs reshape data interpretation, they enable organizations to uncover intricate relationships within their data, enhancing overall strategic alignment.
Moreover, understanding the integration of knowledge graphs with URL shorteners and link management systems can further amplify their value. With URL shorteners optimizing link usage data, combining this with a knowledge graph approach could allow businesses to analyze link performance dynamically. Such integrations can lead to more tailored marketing strategies and deeper analytics, ensuring that digital professionals remain ahead of the curve in the competitive landscape.
Conclusively, the promise of knowledge graphs as a powerful analytical mechanism cannot be understated. As industries evolve, these graphs will emerge as indispensable tools that facilitate a nuanced comprehension of complex information ecosystems.
#BitIgniter #LinksGPT #UrlExpander #UrlShortener #KnowledgeGraph #DigitalMarketing #DataScience
Want to know more: Learn more